Shrink Your VA Model Neural Networks!
Capturing the complex nonlinear behaviors of analog circuits in virtual analog modeling is a significant challenge. Selecting the appropriate neural network size and architecture for these function approximation tasks currently relies heavily on trial-and-error methods like grid search. These approaches are time-consuming, computationally intensive, and lack a solid theoretical foundation, often resulting in oversized models that are inefficient and impractical for real-time applications. This talk introduces ideas for a framework that systematically determines optimal neural network architectures for modeling. The talk will speak about examining the geometric structures and symmetries in the complexity of the model, and the designation of neural network architectures that replicate these systems while focusing on efficient resource utilization. This approach centers specifically on function approximation, tailoring the network architecture to the mathematical functions underlying the model. Large networks memorize training data instead of learning underlying patterns, leading to poor generalization; additionally, oversized networks consume excessive computational resources, resulting in increased memory usage, adding to the challenge of hardware limitations and the necessity for low-latency responses.a as code, code as a compiler, and the output as a function. This perspective aims to streamline the development process and enhance the practical deployment of neural networks in audio signal processing.
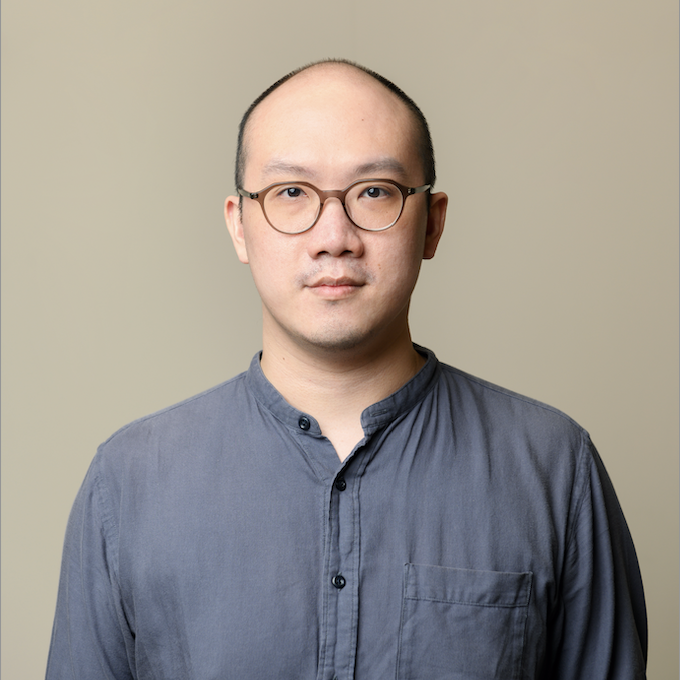
Christopher Clarke
DSP Software Developer (C++)
Graduated PhD in with research in Artificial Intelligence (DSP) from the Singapore University of Technology and Design (Science, Math, and Technology Cluster) – President’s Fellowship Program & Computer and Information Sciences (CIS) Scholarship. Member of the Interdisciplinary Audio and Acoustics Research Group at SUTD. My passion lies with low-latency audio plugin/framework implementations, particularly for applications that have traditionally been deemed otherwise. My PhD's research focuses on the AI/ML technologies to run extremely low-latency (microsecond and below) audio processing, even on low-compute devices such as embedded microcontrollers or System-on-a-Chip. As a Music Technologist with focus on generative algorithms and stochastic modelling for music generation, I have presented fixed site-specific installations and deployed software libraries on music generation