Knee-Deep Learning
Practical Steps to Get Started with Audio ML
Dive in and start creating!
Dive into the basics of machine learning for audio and start creating with a few practical steps.
This talk is aimed at developers without prior experience in machine learning who want to get inspired and equipped with the knowledge to start their own projects. The purpose is to provide a practical introduction to the topic in order to demystify theory and overcome implementation complexities.
Whether you're looking to solve complex problems where traditional DSP methods fall short or conjure up unthinkable sounds, this session is for you.
We dive right in, using simple and free tools to acquire data, set up code to create an ML training and inference pipeline, explore training techniques, and analyze and evaluate the results as we go. We cover what hardware is needed for training at different scales, ranging from cloud computing to consumer GPUs.
We'll cover basic theory, a brief history of different approaches, and, in particular, practical advice on getting started: data requirements, data acquisition, training, hardware needs, and deployment, including options for on-device real-time inference, embedded systems, and cloud-based SaaS.
Throughout, simple example model architectures suitable for beginners are used.
After training and analyzing some simple models, we explore different deployment options, including cloud-based inference, on-device native code using popular inference frameworks, and dedicated embedded hardware modules.
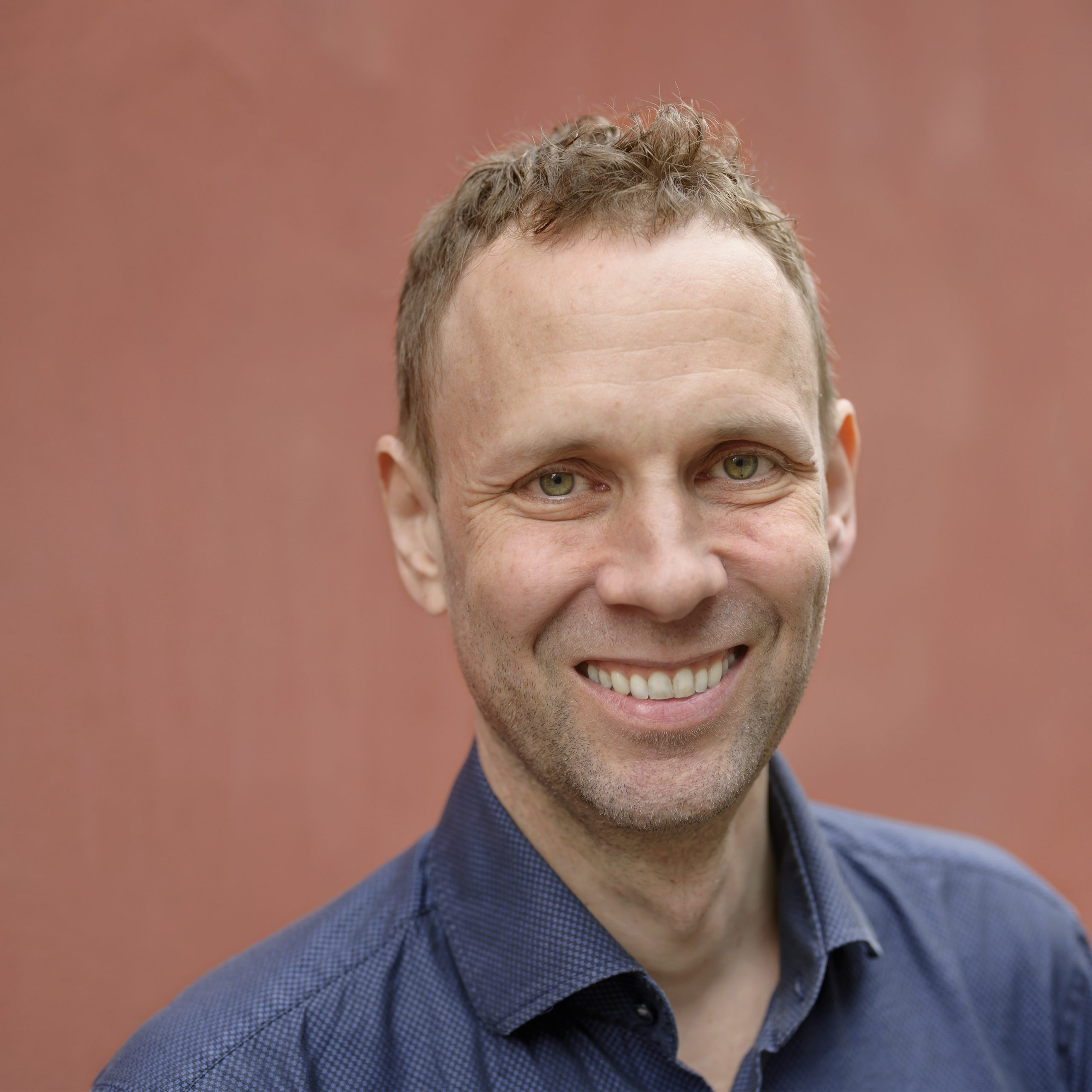
Martin Swanholm
CTO
Hindenburg Systems
Martin is a software developer and DSP engineer with over 30 years of experience, focusing on practical, real-world applications of machine learning in audio. His work emphasizes getting the most out of available hardware and compute resources, ensuring solutions are efficient and accessible to a wide range of users. He is currently developing effective tools for audio restoration, like phase-coherent frequency-domain models and multi-task learning models that improve speech off-line or interactively in real time.
Martin’s journey in digital audio began in the 1990s, and over the years, he’s worked on everything from basic signal processing to full multimedia systems. His approach is rooted in pragmatism—using techniques that work, whether simple or advanced, to solve real problems.
Martin excels at breaking down complex concepts into clear, actionable steps, making his presentations valuable for beginners looking to understand audio processing with machine learning. He’s committed to showing how practical, tried-and-true methods can yield strong results without requiring cutting-edge hardware or expertise, making his sessions approachable for all skill levels.